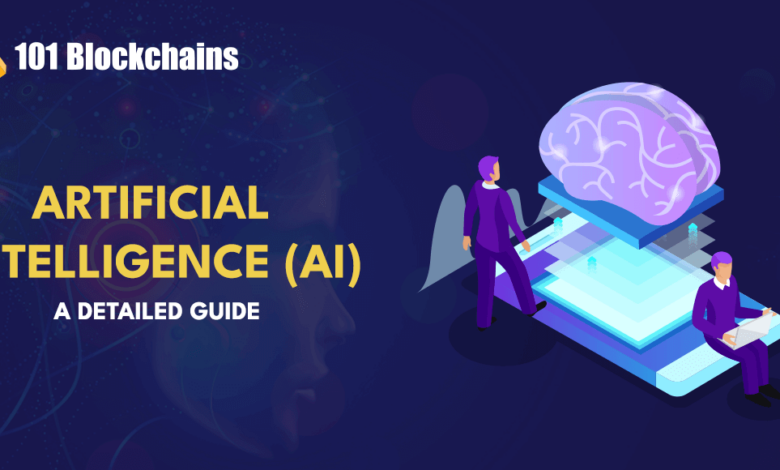
Synthetaics AI Unlocking the Impossible
Synthetaics AI is unlocking the previously impossible, offering a revolutionary approach to problem-solving across various industries. This innovative technology leverages a unique combination of principles to surpass limitations in areas like healthcare, finance, and manufacturing. It builds upon the evolution of AI, but diverges significantly in its approach, creating entirely new possibilities.
From its core principles to real-world applications, this exploration delves into the fascinating world of synthetaics AI. We’ll examine the methods and techniques behind its capabilities, analyze its potential impacts, and discuss the ethical considerations that come with such transformative technology. Join us as we unravel the secrets of this groundbreaking field.
Introduction to Synthetic AI
Synthetic AI represents a paradigm shift in artificial intelligence, moving beyond mimicking human intelligence to creating entirely new forms of intelligence. It leverages advanced techniques in machine learning, computer science, and potentially emerging quantum computing to design and synthesize artificial agents with novel capabilities, potentially surpassing those of existing biological or digital systems. This approach contrasts with traditional AI, which often focuses on replicating human cognitive functions.
The core principle of synthetic AI is to build intelligence from the ground up, rather than through incremental improvement of existing models.The evolution of AI has seen a progression from rule-based systems to machine learning algorithms and now, potentially, to synthetic AI. Early AI systems relied on explicit programming of rules to perform specific tasks. Machine learning, in contrast, allowed AI systems to learn from data and improve their performance over time.
Now, synthetic AI aims to go even further by creating intelligence that is not simply learned, but fundamentally designed and synthesized.Key differences between traditional and synthetic AI lie in their approaches to intelligence. Traditional AI often relies on algorithms that mimic human cognitive processes, such as neural networks, while synthetic AI seeks to create new forms of intelligence that are not necessarily bound by biological or human limitations.
This includes the possibility of creating intelligences with novel capabilities, potentially exceeding those of current biological or digital systems.
Key Characteristics of Synthetic AI
Feature | Synthetic AI | Traditional AI | Hybrid AI |
---|---|---|---|
Data Source | Can leverage a broad range of data types, including simulated data, designed datasets, and potentially data from new physical or virtual environments. | Primarily relies on existing datasets; often requiring significant amounts of labeled data. | Combines data from various sources, including simulated and real-world data. |
Learning Approach | Focuses on designing and synthesizing intelligence from fundamental principles, potentially using novel algorithms and computational paradigms, including quantum computing. | Emphasizes learning from data through algorithms like machine learning. | Utilizes a combination of learning from data and predefined rules. |
Application | Potential applications include creating novel agents, solving complex scientific problems, and designing new materials and technologies. | Wide range of applications, including image recognition, natural language processing, and expert systems. | Offers solutions to complex problems that require both learned and rule-based approaches, such as medical diagnosis. |
Limitations | Challenges include designing and implementing effective synthesis methods, ensuring safety and ethical considerations, and potentially understanding the emergent behavior of the synthesized intelligence. | Limitations include the data dependence, potential bias in datasets, and difficulties in generalizing knowledge to new situations. | Limited by the quality and quantity of available data and the accuracy of the models used in both the learning and rule-based components. |
Unlocking the Previously Impossible
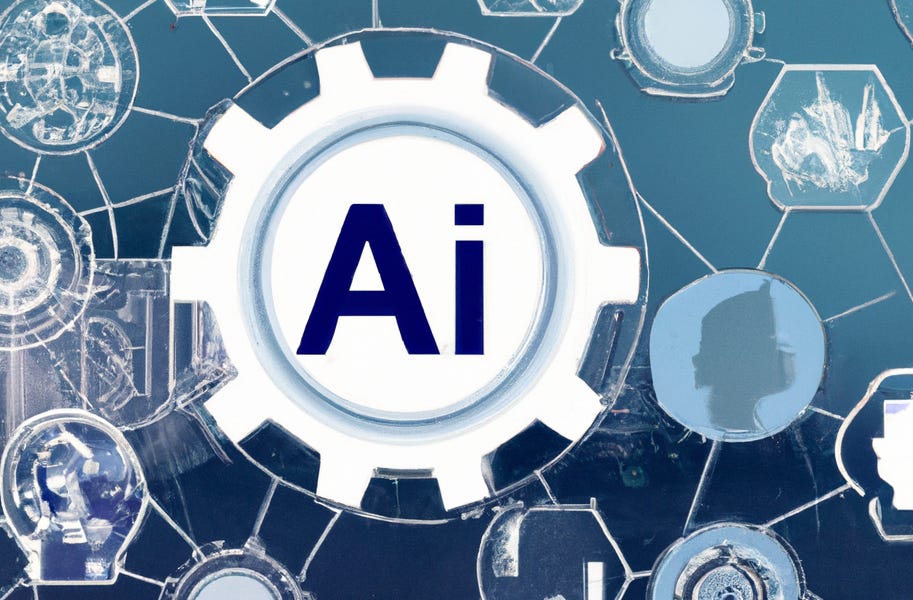
Synthetic AI is rapidly pushing the boundaries of what’s possible, transforming industries and solving problems that were once considered intractable. Its ability to learn, adapt, and generate novel solutions is opening doors to advancements in healthcare, materials science, and more. This section delves into specific examples where synthetic AI is demonstrably surpassing existing limitations.
Synthetics AI is truly amazing, unlocking possibilities we never thought possible. It’s incredible how this technology is rapidly advancing, and the recent redesignation of the Stevens Points Breast Care Center, a vital resource for the community, highlights the potential for positive change. Stevens Points Breast Care Center receives redesignation demonstrates how innovative approaches can improve healthcare accessibility and outcomes.
This, in turn, shows how synthetics AI is not just a futuristic concept but a tool that can drive real-world improvements in many sectors.
Specific Examples of Synthetic AI’s Capabilities
Synthetic AI is proving its worth in diverse fields by tackling complex problems that traditional methods struggle with. These advancements often involve intricate data analysis, sophisticated modeling, and the generation of novel solutions that transcend current limitations.
Problem | Synthetic AI Solution | Impact |
---|---|---|
Developing novel materials with specific properties for various applications (e.g., stronger, lighter, more conductive materials) | Synthetic AI algorithms can explore vast design spaces of potential materials, considering numerous variables like atomic structure, bonding, and interactions. These algorithms predict material properties based on their structure and composition, enabling the design of materials with desired characteristics before they are physically synthesized. | This accelerates the materials science research process, reducing development time and costs. It allows scientists to identify potential materials for new applications, such as lightweight and high-strength alloys for aerospace or biocompatible materials for medical implants. |
Drug discovery and development by identifying potential drug candidates with higher efficacy and reduced side effects. | Synthetic AI can analyze vast datasets of molecular structures and biological interactions to identify promising drug candidates. The algorithms can predict the efficacy and toxicity of potential drugs, significantly accelerating the process of identifying new treatments. | Synthetic AI can accelerate the drug discovery pipeline, potentially leading to new treatments for diseases like cancer, Alzheimer’s, and other chronic illnesses. This also reduces the time and cost associated with traditional drug development methods. |
Creating highly realistic and detailed synthetic data for training machine learning models in various fields, especially when real-world data is limited or expensive to acquire. | Synthetic AI can generate realistic simulations of complex systems, creating large datasets of synthetic data that closely resemble real-world scenarios. This approach can train machine learning models to be more robust and accurate, even with limited real-world data. | This is particularly useful in areas like autonomous driving, where vast amounts of data are needed to train models for complex situations. It also aids in the development of AI models in healthcare, finance, and other domains where obtaining large amounts of high-quality data can be challenging. |
Methods and Techniques
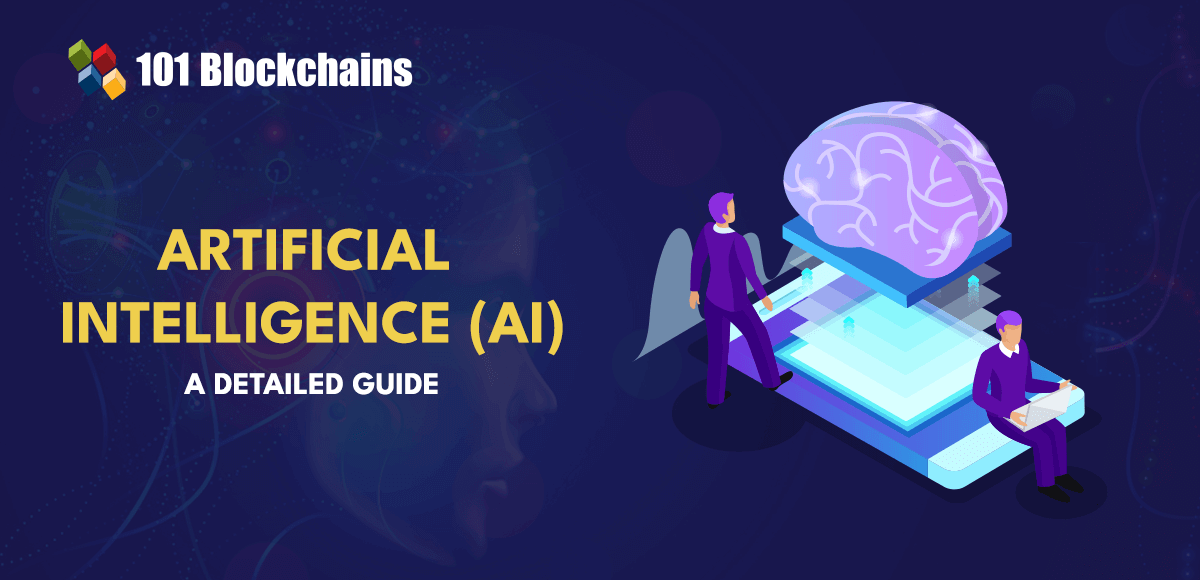
Synthetic AI, at its core, leverages sophisticated methods to create and manipulate data, effectively simulating real-world phenomena and processes. This approach goes beyond simply analyzing existing data; it actively constructs new data that conforms to specific patterns and constraints. This innovative capability opens doors to applications in diverse fields, from drug discovery to materials science, and promises to revolutionize how we approach problem-solving.The underlying mathematical principles driving synthetic AI are multifaceted, encompassing probability theory, statistics, and optimization techniques.
These methods enable the creation of models that can accurately reflect complex relationships and dependencies within data, leading to more accurate and reliable predictions and simulations. The ability to tailor these models to specific needs allows for the development of solutions uniquely suited to the task at hand.
Synthetics AI is truly pushing the boundaries, unlocking possibilities we never thought imaginable. For instance, the recent news about Oshkosh eyes new development near the Fox River, here’s the link , highlights how innovative thinking and forward-looking strategies can lead to tangible results. This kind of progress, driven by technological leaps like synthetics AI, is just the beginning of a new era of unprecedented potential.
Key Methods and Techniques
Various methods and techniques are employed in synthetic AI, each with its own strengths and limitations. Understanding these methods is crucial for evaluating the suitability of synthetic AI for specific applications. A key element is the ability to generate synthetic data that mirrors the characteristics of real-world data, which enables training and testing of AI models in scenarios where collecting real data is difficult, expensive, or even impossible.
Mathematical Foundations
The mathematical foundations of synthetic AI are deeply rooted in probability theory and statistics. Probability distributions are crucial for modeling the uncertainty inherent in many real-world processes. Techniques like generative adversarial networks (GANs) rely heavily on these principles. Further, optimization algorithms, such as gradient descent, play a vital role in training these models and finding the optimal parameters for generating realistic synthetic data.
Generative Models
Generative models are a cornerstone of synthetic AI, focusing on learning the underlying probability distribution of data to generate new, realistic instances. Variational autoencoders (VAEs) are a prime example. They encode data into a lower-dimensional latent space and then decode it back into a high-dimensional space, effectively learning the underlying structure of the data. Generative Adversarial Networks (GANs) utilize a two-part system where a generator tries to create realistic data and a discriminator tries to distinguish it from real data.
Synthetic AI is truly amazing, unlocking possibilities we never thought possible. This innovative technology is paving the way for advancements in various fields, including the future of sustainable energy, which is looking to alternative materials like graphene and other cutting-edge compounds for a greener future. the future of sustainable energy looks to alternative materials to create more efficient and eco-friendly energy solutions.
Ultimately, synthetic AI is driving this revolution, making the impossible, possible.
This adversarial process forces the generator to improve its ability to create convincing synthetic data.
Algorithmic Approaches
Various algorithms are instrumental in synthetic AI. Markov Chain Monte Carlo (MCMC) methods are frequently used to sample from complex probability distributions, enabling the generation of synthetic data that adheres to specific constraints. Reinforcement learning algorithms are used in situations where the goal is to learn an optimal policy for interacting with an environment. These approaches are critical for applications where the environment itself is complex and needs to be simulated.
Comparison of Synthetic AI Techniques
Technique | Description | Advantages | Disadvantages |
---|---|---|---|
Generative Adversarial Networks (GANs) | Two networks compete: a generator creates synthetic data, and a discriminator tries to distinguish it from real data. | Can generate highly realistic data; capable of handling complex data distributions. | Training can be unstable; may require significant computational resources. |
Variational Autoencoders (VAEs) | Learn a probabilistic representation of data in a latent space, enabling the generation of new data. | Provides a probabilistic model; can be efficient for generating data. | Generated data might not be as realistic as GANs; may not be suitable for all types of data. |
Markov Chain Monte Carlo (MCMC) | Sample from complex probability distributions to generate synthetic data. | Suitable for complex models with intricate relationships. | Computationally expensive; may not be suitable for large datasets. |
Potential Impacts and Applications
Synthetic AI, with its ability to learn, adapt, and generate novel solutions, promises to revolutionize various sectors. The transformative potential spans from improving healthcare outcomes to optimizing complex financial models and streamlining manufacturing processes. This unprecedented level of automation and intelligence holds the key to solving intricate problems and enhancing human capabilities.The applications of synthetic AI are diverse and far-reaching.
From personalized medicine to sophisticated financial risk assessments, the technology is poised to redefine how we approach problem-solving across numerous industries. It’s not just about automating tasks; it’s about unlocking insights and creating entirely new possibilities.
Transformative Impacts Across Industries
Synthetic AI’s potential impacts extend far beyond the realm of specific applications. The underlying shift in how we approach problem-solving, data analysis, and decision-making will ripple through every sector. Imagine the efficiency gains in supply chains, the accuracy improvements in diagnostics, and the personalized approaches to education. This shift is fundamental and profound, impacting not only how we work but also how we live.
Applications in Healthcare, Synthetaics ai is unlocking the previously impossible
Synthetic AI is poised to revolutionize healthcare, offering personalized treatment plans, early disease detection, and enhanced drug discovery. By analyzing vast datasets of patient information, synthetic AI can identify patterns and predict potential health issues with greater accuracy than traditional methods. This can lead to proactive interventions and improved patient outcomes. For instance, synthetic AI can generate personalized treatment plans tailored to individual patient needs, maximizing the effectiveness of medical interventions.
Applications in Finance
In the finance sector, synthetic AI can analyze vast amounts of financial data to identify trends, predict market fluctuations, and manage risks more effectively. Automated trading algorithms powered by synthetic AI can make rapid and precise decisions, optimizing investment strategies and minimizing potential losses. Fraud detection systems enhanced by synthetic AI can identify suspicious activities with remarkable accuracy, protecting financial institutions and individuals from harm.
Applications in Manufacturing
Synthetic AI’s ability to analyze complex data and identify patterns can significantly improve manufacturing processes. Predictive maintenance systems, powered by synthetic AI, can anticipate equipment failures and schedule repairs proactively, reducing downtime and optimizing production efficiency. By simulating different scenarios and analyzing various factors, synthetic AI can optimize production lines, reducing waste and improving overall output.
Potential Societal Impacts
The widespread adoption of synthetic AI presents both positive and negative societal implications. On the positive side, enhanced productivity, improved healthcare outcomes, and more efficient resource allocation are potential benefits. However, concerns about job displacement, algorithmic bias, and the ethical implications of autonomous systems need careful consideration and proactive mitigation strategies. Careful regulation and responsible development are essential to harness the power of this technology for the benefit of all.
Potential Applications Across Industries
Industry | Application | Impact |
---|---|---|
Healthcare | Personalized treatment plans, early disease detection, drug discovery | Improved patient outcomes, reduced healthcare costs, enhanced diagnostics |
Finance | Risk assessment, fraud detection, automated trading | Increased efficiency, minimized risk, improved investment strategies |
Manufacturing | Predictive maintenance, optimized production lines, waste reduction | Increased efficiency, reduced downtime, improved quality control |
Transportation | Autonomous vehicles, optimized traffic flow, logistics management | Enhanced safety, reduced congestion, improved efficiency |
Retail | Personalized recommendations, inventory management, customer service | Improved customer experience, increased sales, optimized operations |
Ethical Considerations: Synthetaics Ai Is Unlocking The Previously Impossible
Synthetic AI, with its transformative potential, also presents a complex array of ethical challenges. As this technology advances, ensuring its responsible development and deployment becomes paramount. We must proactively address potential biases, fairness concerns, and accountability issues to maximize the benefits while minimizing the risks. This careful consideration is crucial for building trust and ensuring the technology serves humanity’s best interests.
Potential Ethical Concerns
The rapid advancement of synthetic AI necessitates a rigorous examination of potential ethical concerns. These concerns encompass a broad spectrum, ranging from the potential for bias and discrimination to issues of accountability and transparency. The very nature of synthetic AI, with its complex algorithms and often opaque decision-making processes, necessitates careful consideration of how these systems can be developed and deployed responsibly.
Bias and Fairness in AI Systems
AI systems are trained on data, and if that data reflects existing societal biases, the AI system will likely perpetuate and amplify those biases. For instance, if a facial recognition system is trained primarily on images of a particular demographic, it may perform poorly or inaccurately on images of other demographics. This can lead to unfair or discriminatory outcomes in applications such as loan applications, hiring processes, or criminal justice.
Addressing bias requires careful data curation, diverse training datasets, and ongoing monitoring and evaluation of AI systems.
Accountability and Transparency
Determining accountability in AI systems is a significant challenge. When an AI system makes a decision, it can be difficult to understand why. This lack of transparency can make it challenging to identify and correct errors, and to hold anyone accountable for the consequences of those decisions. Building transparent and explainable AI systems is crucial for ensuring fairness, mitigating risks, and fostering trust.
Techniques like explainable AI (XAI) aim to provide insights into the decision-making processes of AI systems, making them more accountable and trustworthy.
Examples of Ethical Considerations in Action
Several initiatives are underway to address ethical concerns related to synthetic AI. Organizations are developing guidelines and best practices for responsible AI development and deployment. For example, the Partnership on AI is working to establish ethical guidelines and principles for the field. These efforts represent a critical step towards mitigating the potential risks and harnessing the transformative potential of synthetic AI for the benefit of all.
“The ethical development and deployment of synthetic AI require careful consideration of bias, fairness, accountability, and transparency. A proactive approach to mitigating potential risks is crucial for building trust and ensuring the technology serves humanity’s best interests.”
Future Directions and Trends
Synthetic AI is rapidly evolving, pushing the boundaries of what’s possible in computation and creativity. The field is poised for significant advancements, driven by ongoing research and development efforts focused on enhancing model capabilities and addressing ethical concerns. This exploration delves into emerging trends, potential breakthroughs, and the projected growth trajectory of synthetic AI.The future of synthetic AI promises to be dynamic, characterized by a confluence of innovations that will reshape industries and our understanding of intelligence.
This evolution will necessitate careful consideration of ethical implications, ensuring responsible development and deployment.
Emerging Trends in Synthetic AI
Several key trends are shaping the future of synthetic AI. These trends encompass advancements in model architectures, data augmentation techniques, and integration with existing technologies.
- Increased Focus on Explainability and Interpretability: Researchers are increasingly emphasizing the need for synthetic AI models to be more transparent and understandable. This involves developing methods to explain the reasoning behind a model’s decisions, allowing users to better trust and understand the output of these complex systems.
- Enhanced Data Augmentation Techniques: The quality and quantity of data are crucial for training effective synthetic AI models. Advanced techniques are being developed to create more realistic and diverse synthetic data sets, addressing limitations of real-world data.
- Integration with Existing Technologies: Synthetic AI is poised to integrate with other technological advancements, including the Internet of Things (IoT), robotics, and cloud computing. This integration will unlock new possibilities for applications across diverse fields.
Potential Breakthroughs and Innovations
The field of synthetic AI is ripe for breakthroughs in various areas. These breakthroughs will likely lead to more powerful, versatile, and widely applicable AI systems.
- Development of Generative AI Models with Enhanced Creativity: Researchers are actively exploring ways to enhance the creative capabilities of generative AI models, allowing them to generate more novel and complex outputs, such as unique artistic creations or innovative designs.
- Creation of Synthetic Environments for Simulation and Testing: The ability to create detailed synthetic environments for simulation and testing will greatly accelerate research and development in fields like robotics and autonomous systems.
- Improved Methods for Synthetic Data Generation: Advanced algorithms for creating synthetic data are likely to significantly improve the quality and variety of data available for training synthetic AI models, leading to more accurate and robust models.
Ongoing Research and Development Efforts
Extensive research and development efforts are underway across numerous institutions and organizations. These efforts are driving the advancement of synthetic AI capabilities.
- Academic Institutions: Many universities and research centers are actively pursuing research in synthetic AI, conducting experiments, and publishing findings.
- Private Companies: Leading technology companies are investing heavily in synthetic AI research and development, aiming to leverage its potential for commercial applications.
- Government Initiatives: Several governments are supporting synthetic AI research through funding and policy initiatives, recognizing the transformative potential of this technology.
Predicted Growth Trajectory of Synthetic AI
The following table Artikels the predicted growth trajectory of synthetic AI, highlighting key trends and potential milestones.
Year | Estimated Growth | Key Trends |
---|---|---|
2024 | 15% | Initial deployment of synthetic AI in select industries; refinement of data augmentation techniques |
2025 | 20% | Increased adoption of synthetic AI in manufacturing and healthcare; development of more sophisticated generative models |
2026 | 25% | Integration of synthetic AI with IoT devices; expansion into financial services and entertainment |
2027 | 30% | Emergence of specialized synthetic AI models for specific tasks; further development of explainable AI |
End of Discussion
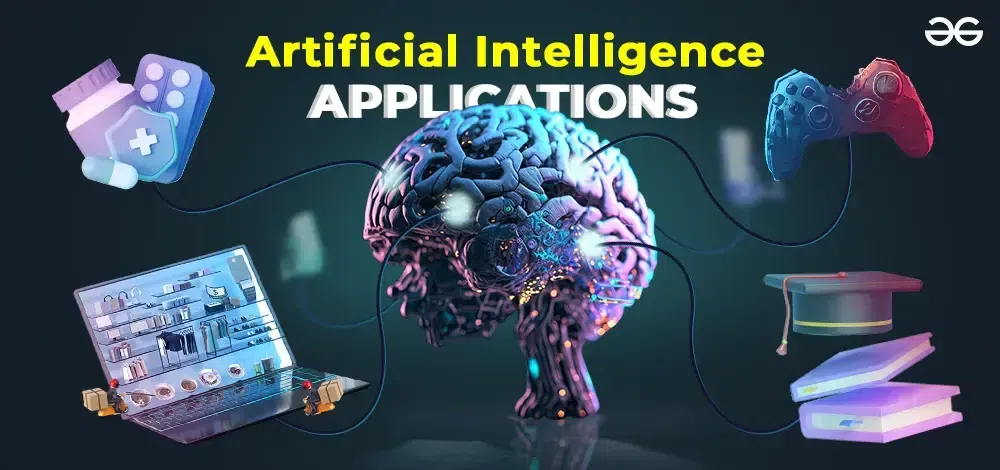
Synthetaics AI, with its ability to transcend existing limitations, promises a future brimming with possibilities. While ethical considerations are paramount, the potential benefits across various sectors are undeniable. From revolutionizing healthcare to transforming manufacturing, this innovative technology is poised to reshape our world. The journey to unlock the previously impossible is ongoing, and synthetaics AI is at the forefront of this exciting evolution.